Ethics in Machine Learning
As machine learning (ML) algorithms become increasingly sophisticated and their applications expand into various domains, concerns about their ethical implications have grown. Ethics in ML encompasses a broad range of issues, including fairness, accountability, transparency, privacy, and societal impact.
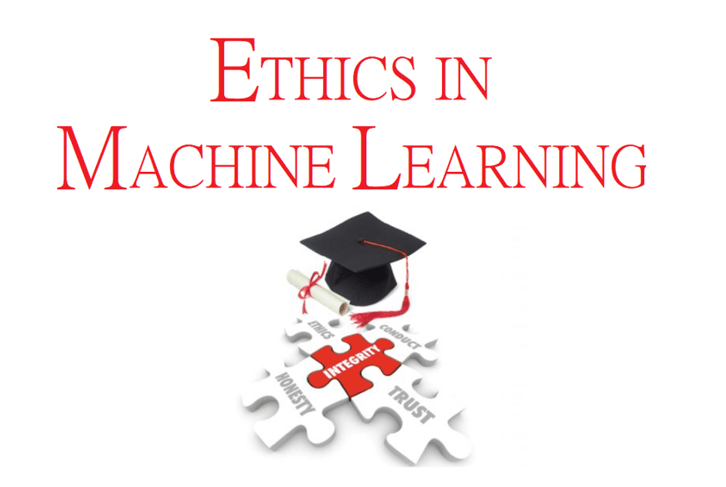
Fairness in Machine Learning
Fairness in Machine Learning refers to the idea that the model's predictions should not be unfairly biased against certain groups of people. Bias can be introduced into the model through the training data, which may reflect historical or societal prejudices. For instance, if a model used for loan applications is trained on data that disproportionately excludes minorities from receiving loans, the model may perpetuate this bias and make discriminatory decisions.
Accountability in Machine Learning
Accountability in Machine Learning refers to the need to identify who is responsible for the decisions made by ML systems. This is particularly important when ML systems make consequential decisions, such as in healthcare or criminal justice. It is crucial to establish clear lines of responsibility to ensure that someone can be held accountable for the system's outcomes.
Transparency in Machine Learning
Transparency in Machine Learning refers to the need to make the inner workings of ML models understandable. This is important for several reasons: it allows for debugging and error correction, it helps to identify potential biases, and it enables users to make informed decisions based on the model's outputs.
Privacy in Machine Learning
Privacy in Machine Learning refers to the need to protect the privacy of individuals whose data is used to train and test ML models. This includes safeguarding sensitive information, such as Personally Identifiable Information (PII), and ensuring that data collection and usage are ethical and compliant with data privacy regulations.
Addressing ethical concerns in ML requires a multi-pronged approach involving researchers, practitioners, policymakers, and the public. It is essential to develop and implement ethical guidelines, promote transparency and accountability, and educate stakeholders about the ethical implications of ML. As ML continues to evolve, ethical considerations must remain at the forefront to ensure that these powerful tools are used responsibly and for the benefit of society.